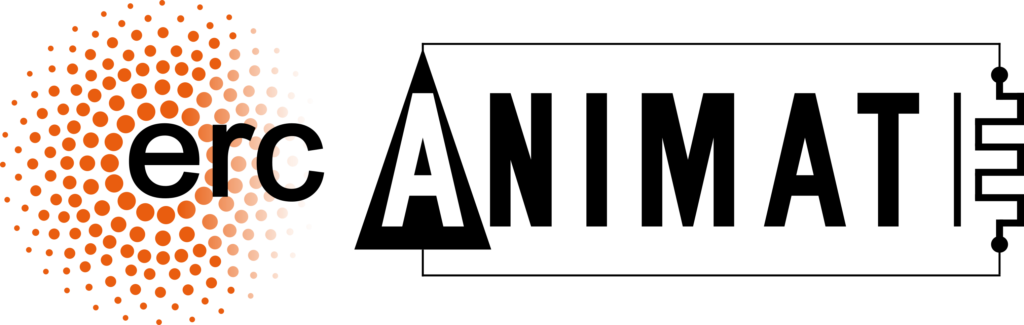
Vast amounts of digital data are processed every day, resulting in high energy consumption. Data centres expend approximately 200 terawatt hours a year, equal to 1 % of global energy demand. Funded by the European Research Council, the ANIMATE project proposes to create a closed-loop in-memory computing model that will be far more energy-efficient because it can perform linear algebraic calculations in just one computational step. The necessary device technology, circuit topologies, system architectures and application portfolio will be developed to validate the model. This model is anticipated to have energy needs that are 5 000 times lower than those of its first-rate digital counterparts.
Objective
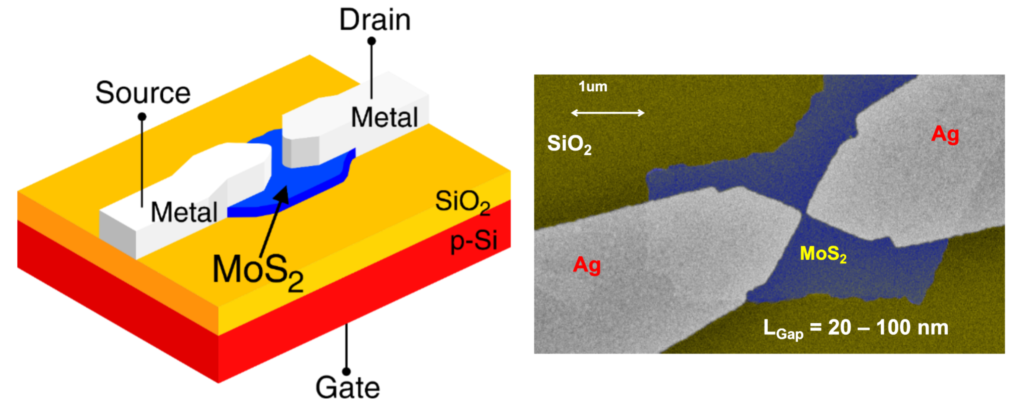
Every day we generate, process and use a massive amount of data. Searching a keyword on the internet, choosing a movie for the weekend and booking our next holiday are just a few simple actions that rely on data-intensive algorithms in the cloud, such as data search, recommendation and page ranking. The energy cost of computation is high: it has been recently reported that training a relatively large neural network produces the same carbon dioxide of 5 cars in their whole lifetime. Data centres use an estimated 200 terawatt-hours each year, corresponding to 1% of the global demand. With the spectre of an energy-hungry future, it is essential to identify novel concepts, novel algorithms and novel hardware for streamlining the computing process.
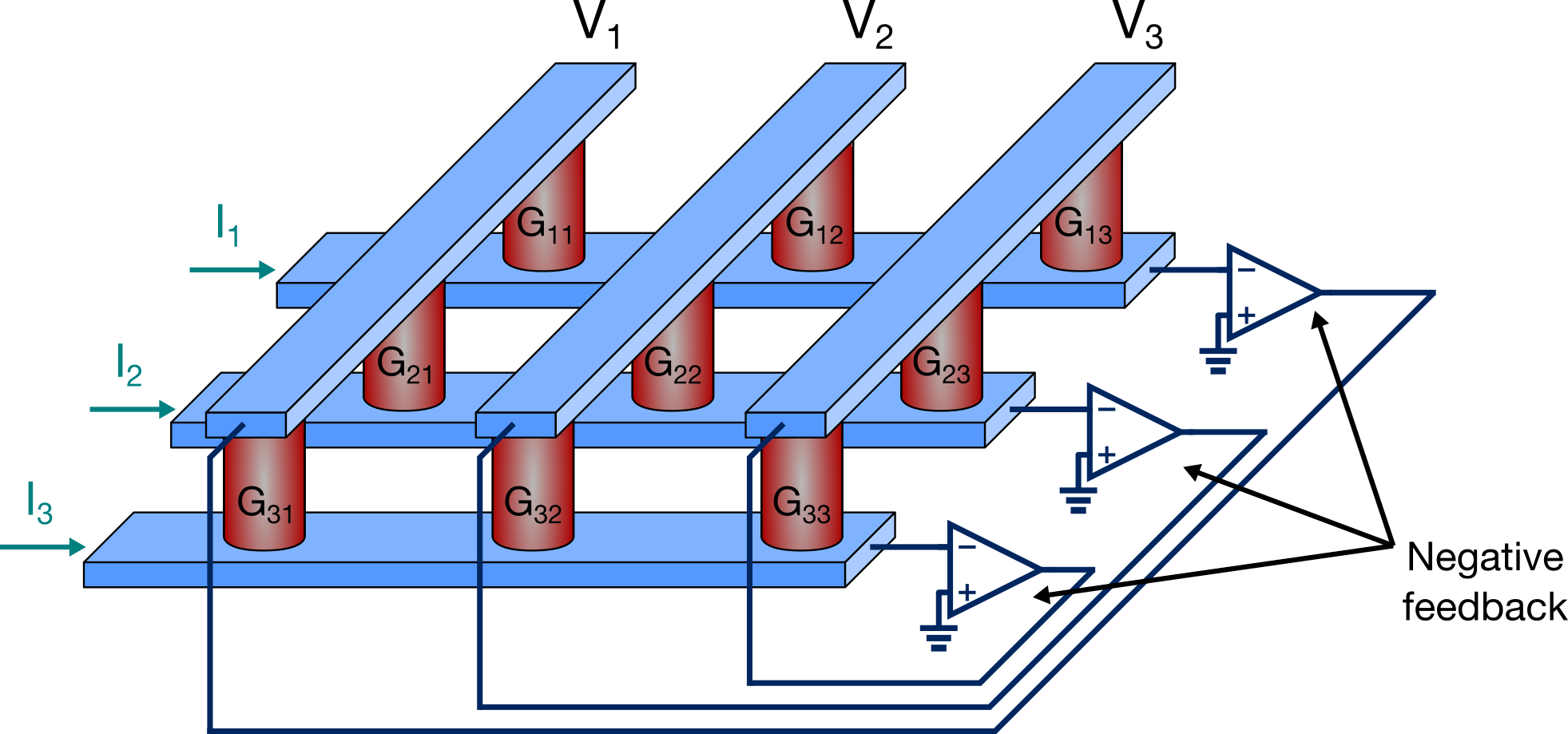
Our preliminary research has shown that computing energy requirements can be reduced by closed-loop in-memory computing (CL-IMC) that can solve linear algebra problems in just one computational step. In CL-IMC, the time to solve a certain problem does not increase with the problem size, in contrast to other computing concepts, such as digital and quantum computers. Thanks to the size-independent computing time around 100 ns, CL-IMC requires 5,000 times less energy than top-class digital computers at the same bit precision. These preliminary results show that CL-IMC is a promising new computing concept to reduce the energy consumption of data processing.
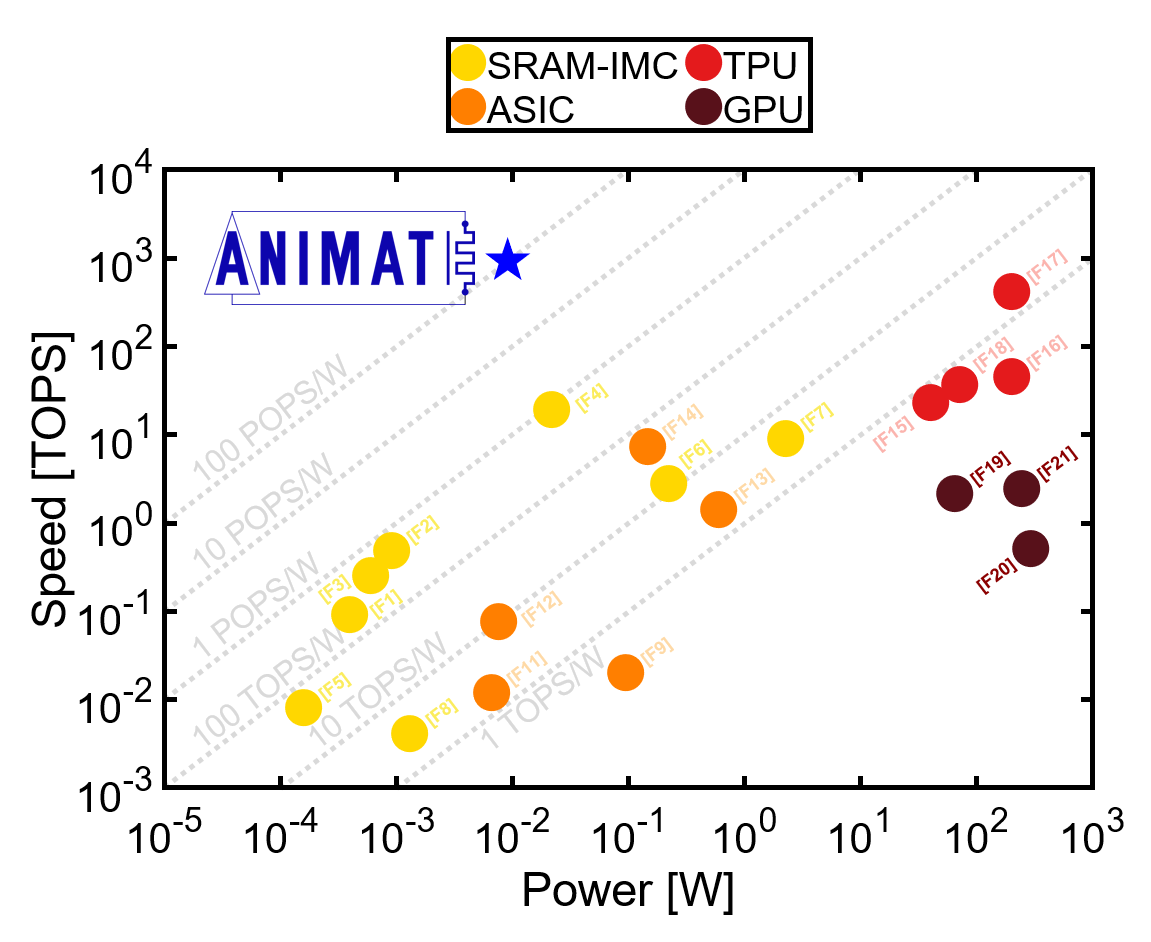
Our project will develop the device technology, the circuit topologies, the system-level architectures, and the application portfolio to fully validate the CL-IMC concept. A novel memory technology that is immune to wire resistance effects will be developed. CL-IMC integrated circuits will be designed with standard CMOS technology. System-level architecture and application exploration will further support the scalability and feasibility of the concept, to demonstrate CL-IMC as a primary contender among the computing technologies with improved energy efficiency.
People
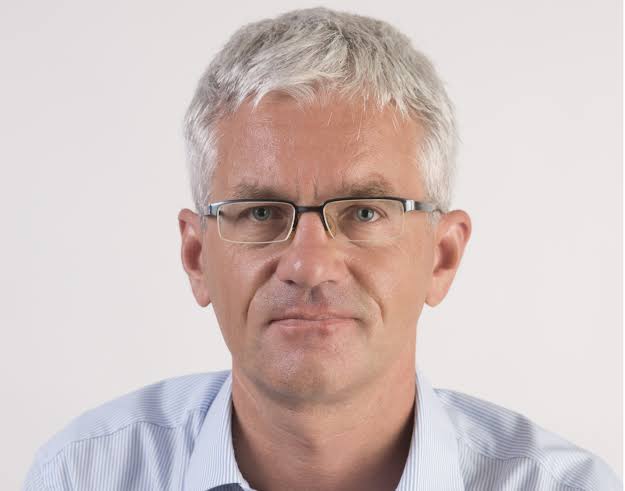
Prof. Daniele Ielmini, Principal Investigator
Prof. Daniele Ielmini received the Laurea (with merit) and Ph.D. in Nuclear Engineering from Politecnico di Milano in 1995 and 2000, respectively. He held visiting positions at Intel Corporation (2006), Stanford University (2006), and the University of Illinois at Urbana-Champaign (2010). His research interests include the modeling and characterization of non-volatile memories, such as nanocrystal memory, charge trap memory, phase change memory (PCM), resistive switching memory (RRAM), and spin-transfer torque magnetic memory (STT-MRAM).
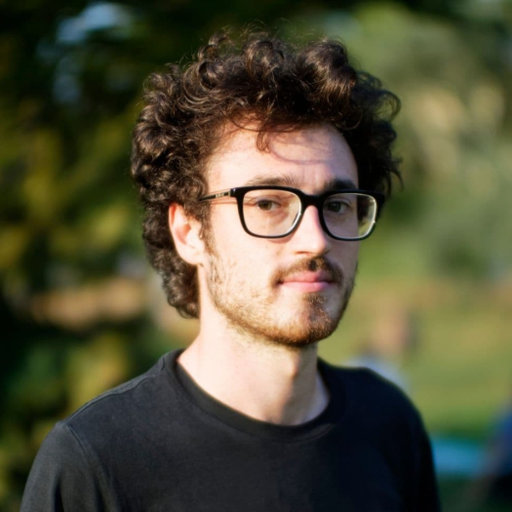
Matteo Farronato, Post-Doc Researcher
Matteo Farronato received his B.Sc, M.Sc., and Ph.D. degrees in Electronics Engineering in Politecnico di Milano in 2017, 2019, and 2023, respectively. During his PhD, he worked on experimental synaptic devices based on 2-dimensional MoS2 semiconductors. Today, he is a post-doc researcher at Politecnico di Milano. His main research interest is the development of new devices for emerging memories and neuromorphic computing.
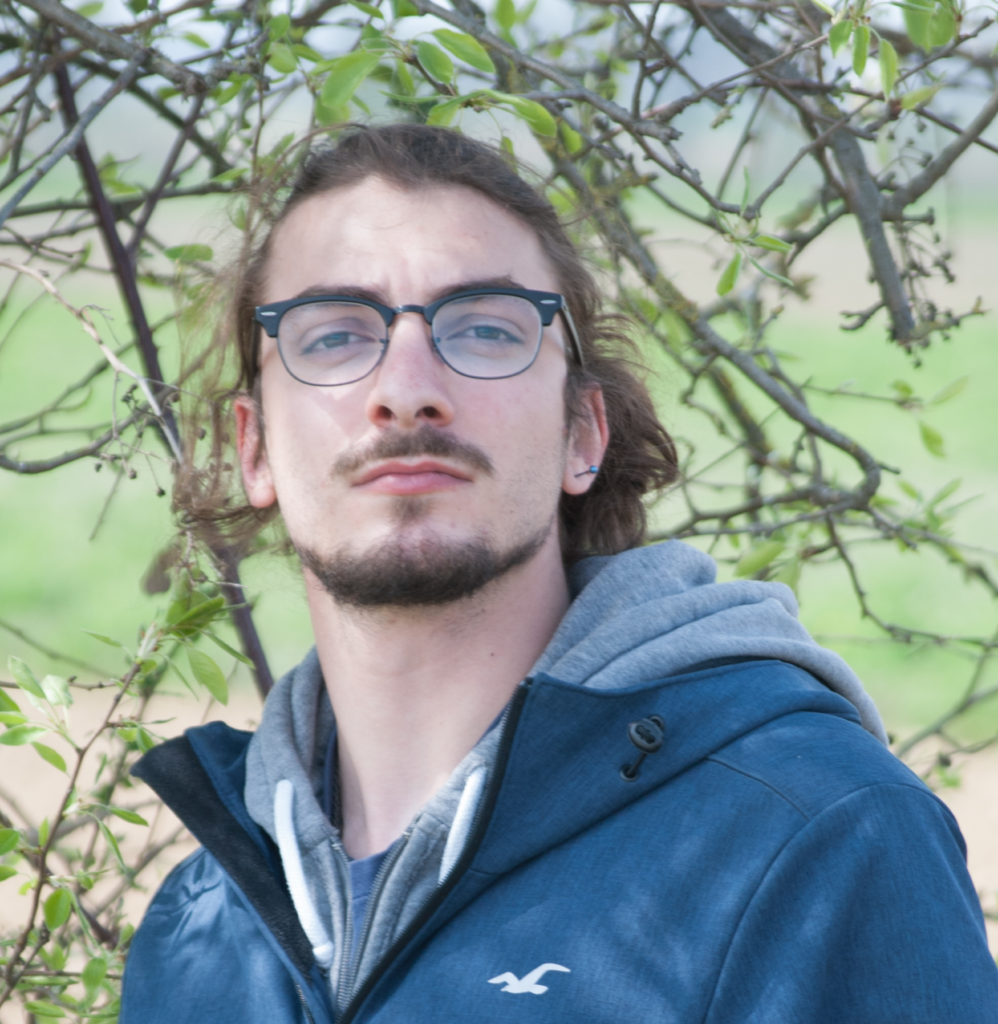
Piergiulio Mannocci, Post-Doc Researcher
Piergiulio Mannocci received the B.Sc., M.Sc., and Ph.D. degrees in Electronics Engineering from Politecnico di Milano in 2016, 2020, and 2023 respectively. From 2017 to 2019, he was Integrated Project Team Leader of the Electronics Team in Skyward Experimental Rocketry. Today, he is a post-doc researcher at Dipartimento di Elettronica, Informazione e Bioingegneria, Politecnico di Milano, working on analog circuit design for in-memory computing with emerging devices.
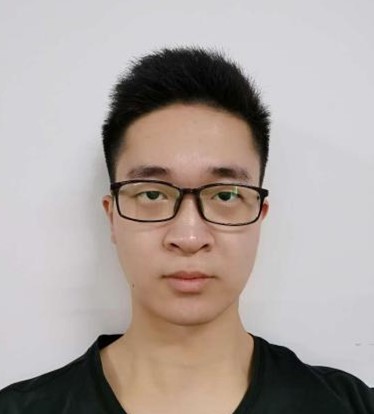
Zirui Chen, Visiting PhD Student
Zirui Chen received the M.Sc. degree in Microelectronics Science and Engineering from Huazhong University of Science and Technology, China, in 2020, where he is currently pursuing the PhD in Electronic Science and Technology. In 2024, he joined the group of prof. Daniele Ielmini as a visiting PhD student, working on closed-loop in-memory computing circuits for compressed sensing.
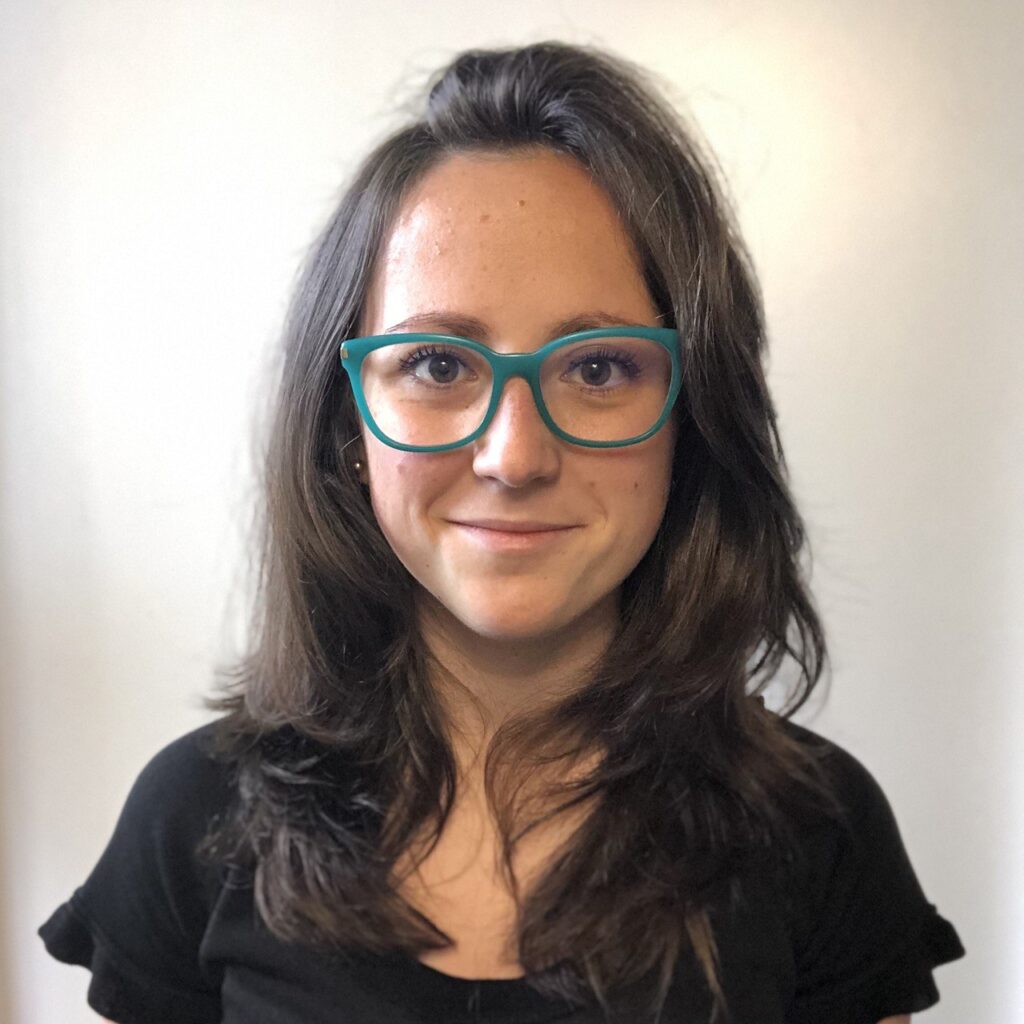
Letizia Pistolesi, PhD Student
Letizia Pistolesi received her B.Sc. and M.Sc. degrees in Electronics Engineering from Politecnico di Milano in 2020 and 2023 respectively. Today she is actively pursuing the PhD in Information Technology at Dipartimento di Elettronica, Informazione e Bioingegneria of Politecnico di Milano working primarily on phase change memory (PCM) for in-memory computing (IMC) accelerators.
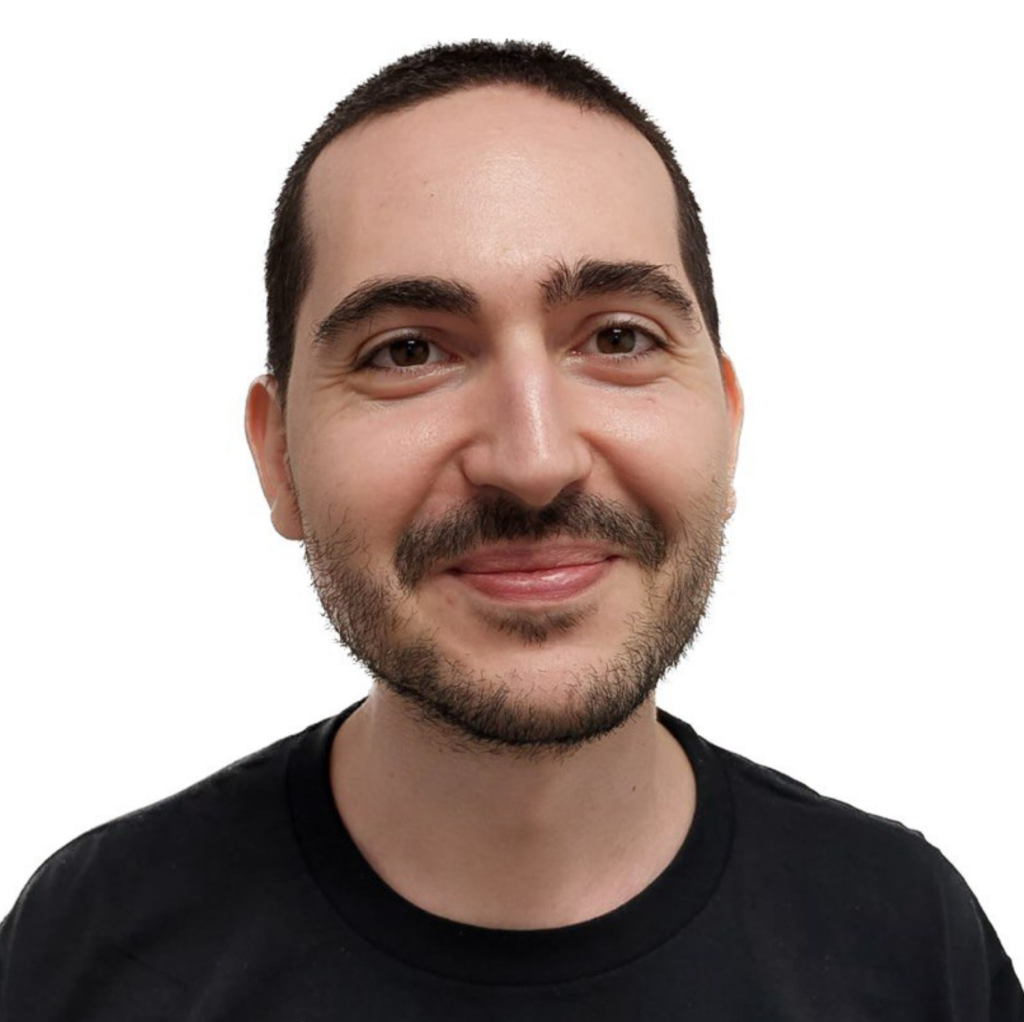
Fabio Carletti, PhD Student
Fabio Carletti received the B.Sc. and M.Sc. degrees in Electronics Engineering from Politecnico di Milano in 2020 and 2023 respectively. Today he is actively pursuing the PhD in Information Technology at Dipartimento di Elettronica, Informazione e Bioingegneria of Politecnico di Milano working primarily on bidimensional memristive devices and 3DXP technologies.
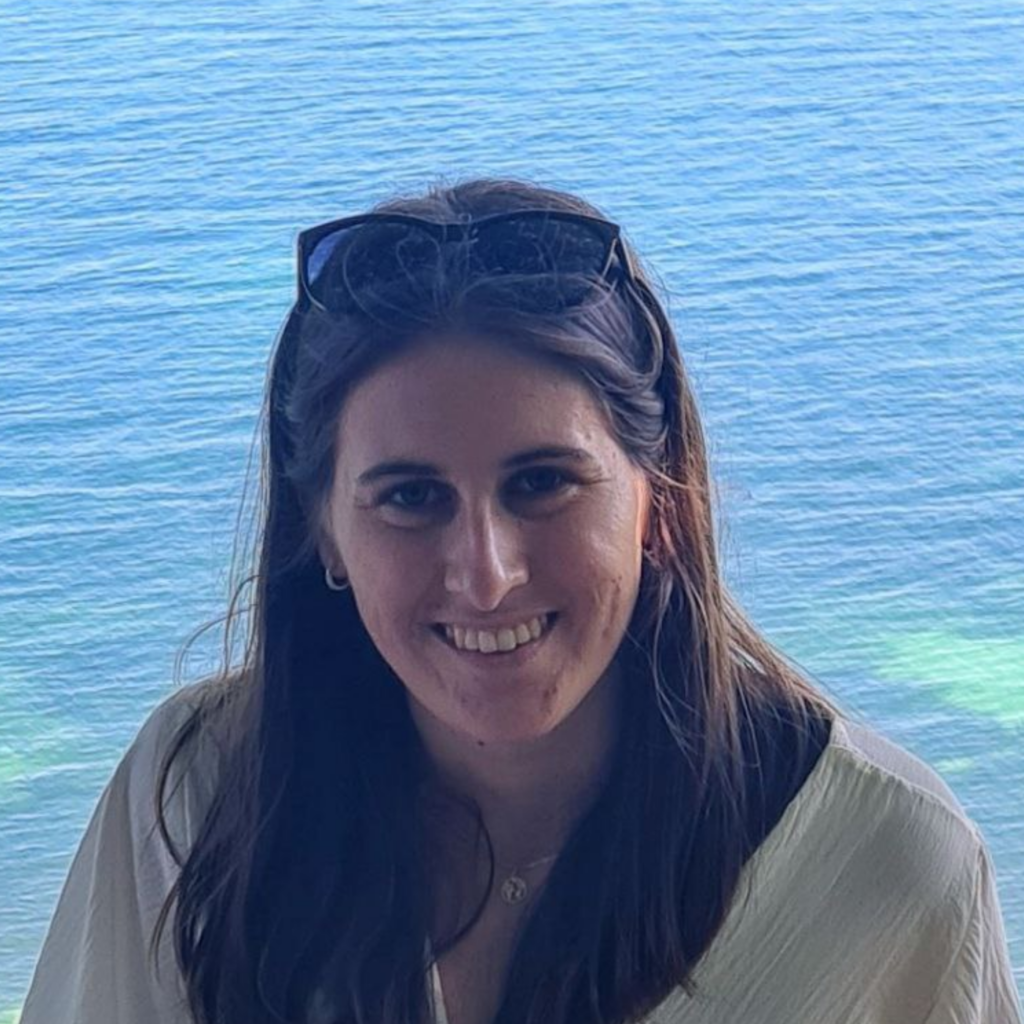
Irene Andreoli, M.Sc. Thesis Student
Irene Andreoli received the B.Sc. degree in Electronics Engineering from Politecnico di Milano in 2022. Today, she is a M.Sc. Thesis student at Dipartimento di Elettronica, Informazione e Bioingegneria, Politecnico di Milano, working on closed-loop in-memory acceleration of inverse kinematics.
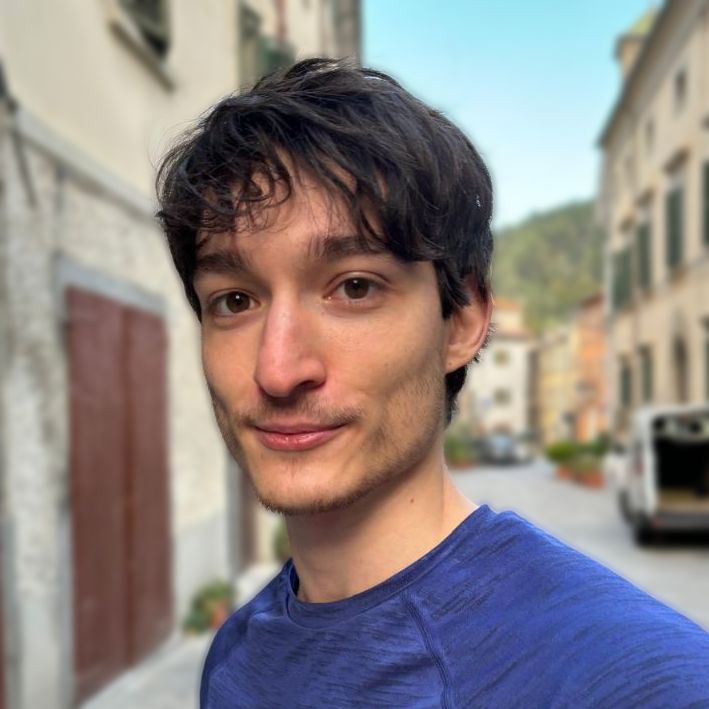
Carlo Zucchelli, M.Sc. Thesis Student
Carlo Zucchelli received the B.Sc. degree in Eletronics Engineering from Politecnico di Milano in 2022. Today, he is a M.Sc. Thesis student at Dipartimento di Elettronica, Informazione e Bioingegneria, Politecnico di Milano, working on novel algorithms for large-scale compilation on closed-loop in-memory computing hardware.
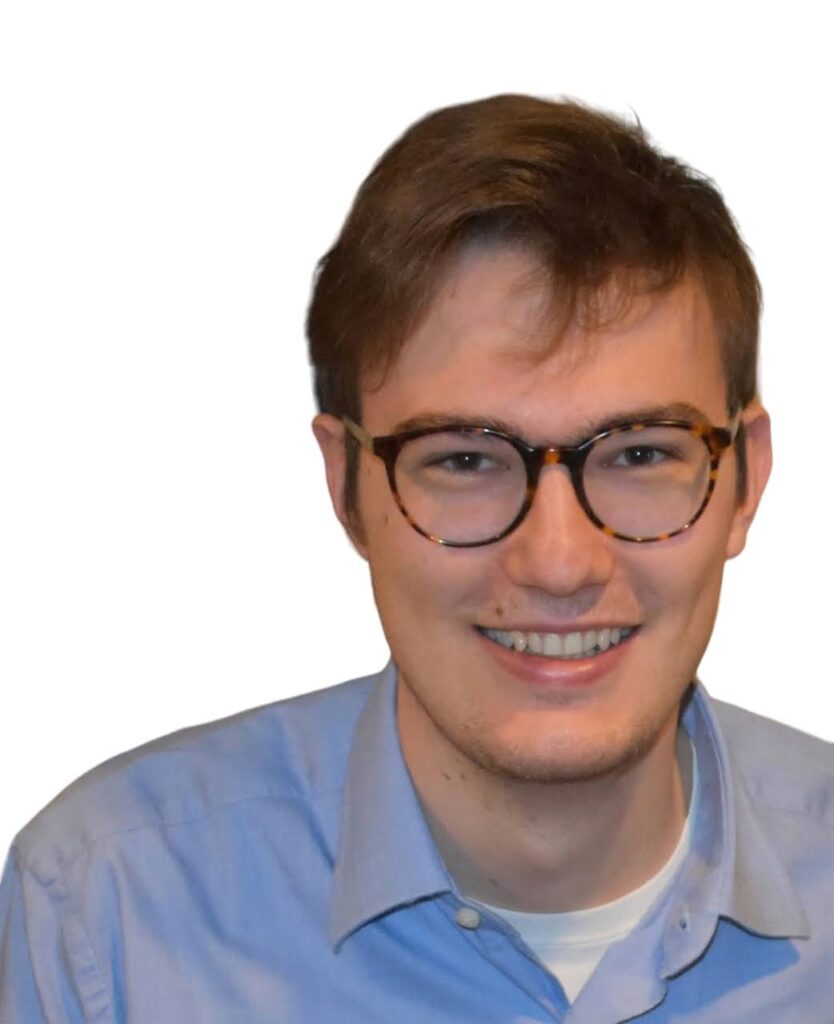
Niccolò Garegnani, M.Sc. Thesis Student
Niccolò Garegnani received the B.Sc. degree in Eletronics Engineering from Politecnico di Milano in 2022. Today, he is a M.Sc. Thesis student at Dipartimento di Elettronica, Informazione e Bioingegneria, Politecnico di Milano, working on modeling and characterization of MoS2-based devices.
Publications
L. Pistolesi, A. Glukhov, A. de Gracia Herranz, M. Lopez-Vallejo, M. Carissimi, M. Pasotti, P. Rolandi, A. Redaelli, I. Muñoz Martin, S. Bianchi, A. Bonfanti, D. Ielmini, “Drift compensation in multilevel PCM for in-memory computing accelerators”, 2024 International Reliability Physics Symposium (IRPS), Dallas, TX, USA, 2024. (Accepted)
P. Mannocci, E. Giannone, D. Ielmini, “In-Memory Principal Component Analysis by Analogue Closed-Loop Eigendecomposition”, IEEE Transactions on Circuits and Systems II: Express Briefs, 2023 (In press). doi: 10.1109/TCSII.2023.3334958
P. Mannocci, E. Melacarne, A. Pezzoli, G. Pedretti, C. Villa, F. Sancandi, U. Spagnolini, D. Ielmini, “An SRAM-based reconfigurable analog in-memory computing circuit for solving linear algebra problems”, 2023 IEEE International Electron Devices Meeting (IEDM), San Francisco, CA, USA, 2023. doi: 10.1109/IEDM45741.2023.10413724.
Z. Chen, Y. Zhou, H. Xu, Y. Fu, Y. Li, Y. He, X.-S. Miao, P. Mannocci, D. Ielmini, “An Ultrafast (< 200 ns) Sparse Solution Solver made by HfWOx/VOy Threshold Tunable Neurons”, 2023 IEEE International Electron Devices Meeting (IEDM), San Francisco, CA, USA, 2023. doi: 10.1109/IEDM45741.2023.10413700
P. Mannocci, E. Giannone, D. Ielmini, “Closed-Loop In-Memory Computing for Energy-Efficient Matrix Eigendecomposition”, 2023 IEEE International Conference on Metrology for eXtended Reality, Artificial Intelligence and Neural Engineering (MetroXRAINE), Milano, Italy, 2023. doi: 10.1109/MetroXRAINE58569.2023.10405821
P. Mannocci, D. Ielmini, “A Generalized Block-Matrix Circuit for Closed-Loop Analog In-Memory Computing”, IEEE Journal on Exploratory Solid-State Computational Devices and Circuits, 9 (1), pp. 47-55, 2023. doi: 10.1109/JXCDC.2023.3265803