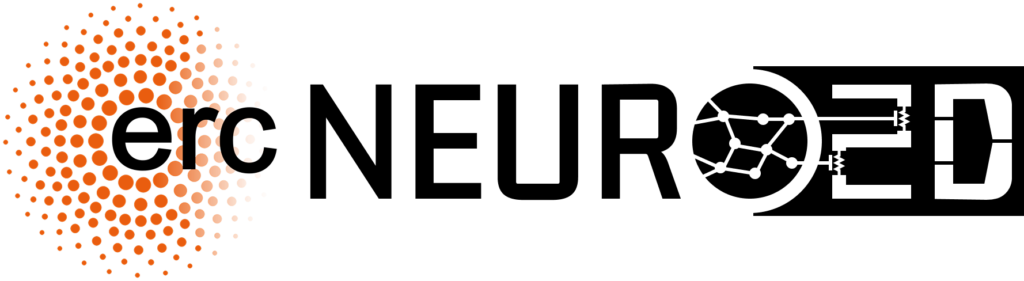
The detection and classification of electrophysiological signals (EPSs), such as electroencephalography (EEG) and electromyography (EMG) recordings, are the gold
standard in neuroscience, enabling the identification of digital biomarkers capable of
health monitoring, personalized medicine and advanced brain-computer interfaces (BCIs). The state-of-the-art technology in this field, however, still relies on bulky, inefficient microelectronic systems which relies on artificial intelligence (AI) in the cloud. The energy efficiency and classification accuracy can be largely improved by neuromorphic computing with emerging materials and devices capable of mimicking the neural mechanisms in our brain. NEURO2D aims at developing a novel class of neuromorphic systems based on reservoir computing (RC) in MoS2, a 2D semiconductor serving as the active material in a charge trap memory (CTM). The device is able to extracted features from EPSs at extremely low power and high accuracy of classification, thus providing efficient digital biomarkers for medical diagnosis and BCIs, booming markets worldwide led by a strong demand for minimally invasive solutions.
Objectives
The NEURO2D project proposes a low-power, high-accuracy neuromorphic technology
based on MoS2 for real-time on-chip analysis of EPSs. MoS2 acts as a reservoir layer for extracting meaningful features, e.g., the salient information, from the raw EPS, thus enabling data compression and on-chip classification with low power, high accuracy, and low latency.
People
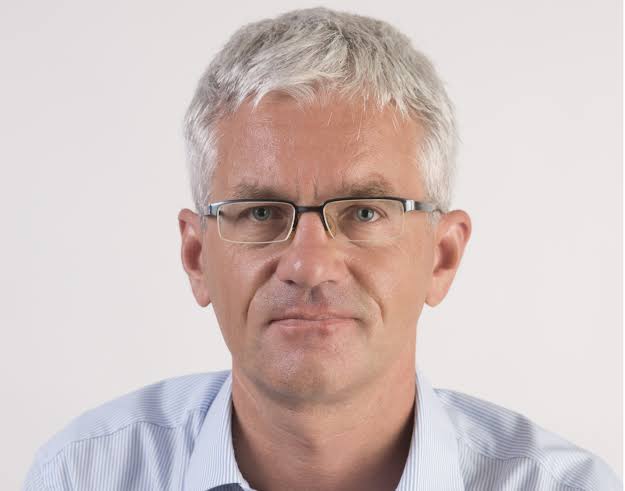
Prof. Daniele Ielmini, Principal Investigator
Prof. Daniele Ielmini received the Laurea (with merit) and Ph.D. in Nuclear Engineering from Politecnico di Milano in 1995 and 2000, respectively. He held visiting positions at Intel Corporation (2006), Stanford University (2006), and the University of Illinois at Urbana-Champaign (2010). His research interests include the modeling and characterization of non-volatile memories, such as nanocrystal memory, charge trap memory, phase change memory (PCM), resistive switching memory (RRAM), and spin-transfer torque magnetic memory (STT-MRAM).
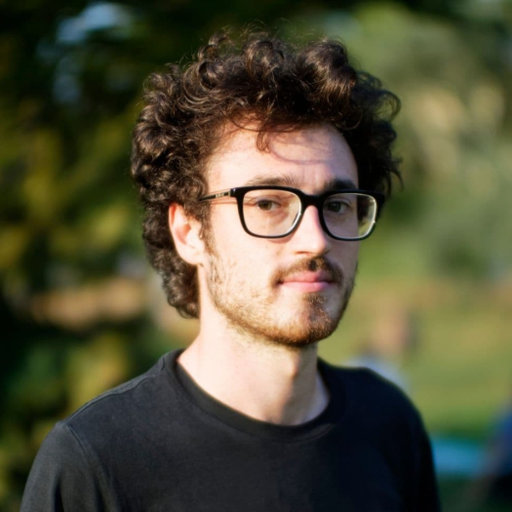
Matteo Farronato, Researcher
Matteo Farronato received his B.Sc, M.Sc., and Ph.D. degrees in Electronics Engineering in Politecnico di Milano in 2017, 2019, and 2023, respectively. During his PhD, he worked on experimental synaptic devices based on 2-dimensional MoS2 semiconductors. Today, he is a post-doc researcher at Politecnico di Milano. His main research interest is the development of new devices for emerging memories and neuromorphic computing.
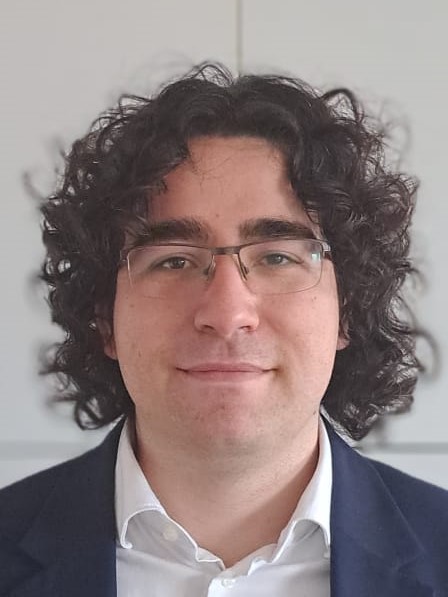
Lorenzo Pittoni, PhD Student
Lorenzo Pittoni received his B.Sc and M.Sc. in Biomedical engineering in Politecnico di Milano in 2021 and 2023, respectively. He is now working on simulations of reservoir computing systems low power signal processing.
Publications
Farronato, M. et al. Seizure detection via reservoir computing in MoS2 -based charge trap memory devices. Sci. Adv. 11, eadr3241 (2025).